Since Renaissance Technologies was founded by James Simons in 1982, AI has been introduced in the finance industry. The historical development of AI focused on logic-based algorithms and Bayesian statistics in the 1960s would later contribute to machine learning techniques used in finance today.
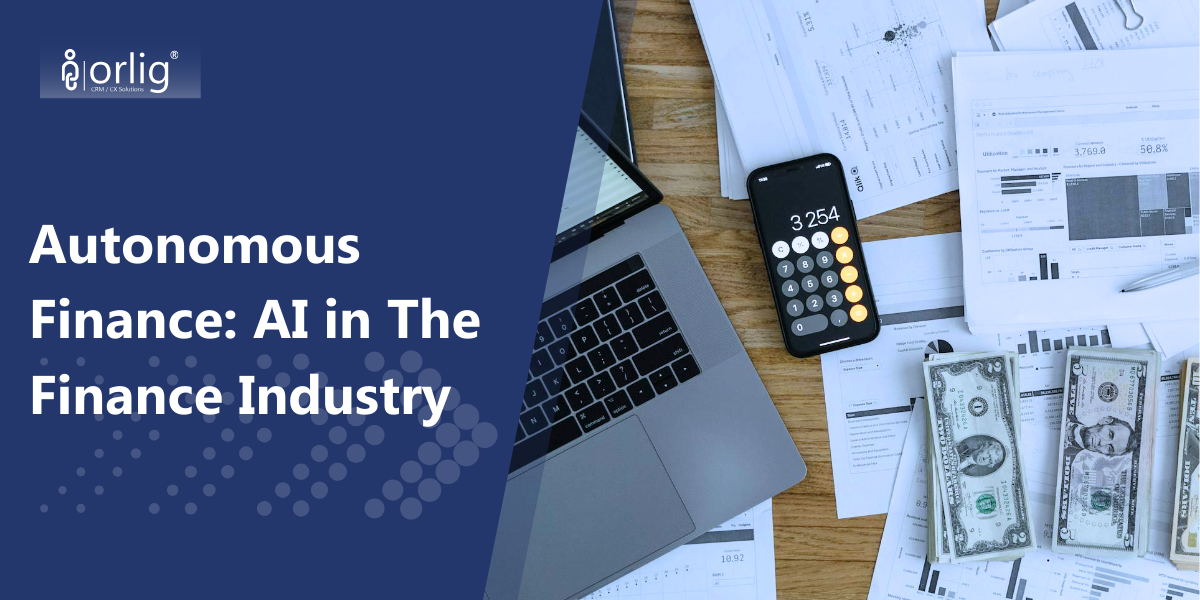
Autonomous Finance
Autonomous finance uses advanced technology, such as AI and ML, to streamline the financial process.
Unlike traditional systems, which heavily rely on human intervention, autonomous finance systems leverage algorithms and data-driven insights to optimise and streamline various aspects of personal and business finance.
Gartner surveyed 64% of CFOs who expected their finance department to be fully automated in the next few years, including the automation of the accounting and financial process, analytics, and real-time and predictive insights.
The Technology of Autonomous Finance
AI/ML
AI (Artificial Intelligence) algorithms analyse data from various sources, enabling the system to identify trends, predict future outcomes, and offer personalized recommendations. This allows financial systems to learn from historical data, identify patterns, and make predictions, allowing the system to adapt to user behaviour, preferences, and market conditions.
ML (Machine Learning) algorithms identifies patterns and trends in financial data, assisting users to make informed financial decisions. By adapting to user behaviour and market conditions, the system can provide real-time recommendations and adjust financial plans accordingly.
An example would be Zest AI, an AI-powered underwriting platform that assists companies in assessing borrowers with little to no credit information or history. The AI uses thousands of data points to provide the transparency that assists lenders in better-assessing populations considered “at risk.” The company reports that auto lenders who use machine-learning underwriting cut losses by 23% annually, more accurately predicted risk and reduced loss by more than 25%.
Blockchain
Blockchain technology revolutionizes financial transactions through transparency and trust as each transaction is securely recorded on the blockchain, linking to previous transactions to form an interchangeable information chain. This eliminates the need for intermediaries and reduces fraud risks, building trust among participants and ensuring the integrity of the financial process.
The benefits of blockchain include:
- Information Management – A disrupted ledger is the core component of blockchain technology, allowing the organization a more accurate and efficient flow of information. This gives the business better insights and more prudent decision-making in the foundation of trust.
- Agility – Blockchain allows an organization to improve its agility as it breaks down silos, creating a network-based system. Reallocating capital based on changing business needs is a top priority for finance leaders as blockchain provides real-time data.
Benefits of Autonomous Finance
Better CX (Customer Experience)
Statistics show that 87% of financial institutions believe that autonomous finance is the key to improving CX, as 66% of today’s customers expect banks to understand their specific needs.
Financial data are obtained and combined with AI-powered autonomous finance as analysing customer behaviours leads to predicting customers’ wants and needs.
Telus International released a report that autonomous finances improve their CX, driving long-term loyalty and customer retention through:
- Simplicity – Autonomous finance requires less manual effort from its customers. This leads to less time spent on performing SWOT analyses and reading product manuals, allowing customers to do more things they enjoy.
- Cost efficiency – An algorithm-driven approach saves customers money by understanding their needs, behaviors, and goals – optimizing their portfolios.
- Proactive support – Autonomous finance unlocks proactive support whereby conversations are prompted when a customer demonstrates certain behaviors, allowing personalization.
Cost & Time Efficient
Autonomous finance saves costs by automating manual processes. It reduces the need for human intervention, especially tasks that require large-scale data analysis that poses a risk of human errors or delayed expense processing that could impact business continuity.
By harnessing algorithms and data analysis, personalized financial planning and automated transactions are provided to enhance efficiency, decision-making, and overall economic well-being.
Financial institutions can better use their customer data with automation as tasks like budgeting, investing, and saving are delegated to automated systems, allowing customers to focus on achieving their financial goals with recommendations based on their preferences and risk tolerance.
Challenges & Considerations of Autonomous Finance
Data Privacy Concerns
AI/ML’s ability to collect and analyse vast amounts of data aids in creating extensive data profiles, allowing the system to predict behaviour, provide personalized services, and offer unique user experiences tailored to each customer’s needs and preferences.
However, the ability to collect, analyse, and predict based on personal data leads to a risk of unauthorized access, misuse, and the potential exploitation of personal information. As autonomous finance relies heavily on data, including personal information such as names, addresses, and financial information, as well as sensitive information such as medical records and social security numbers, there is an inherent risk of data breaches.
These breaches often lead to severe consequences such as:
- Reputational Damage: A data breach can harm the financial agency’s reputation and erode public trust. The incident may result in a loss of credibility, raising concerns about the agency’s ability to safeguard individuals’ personal information.
- Identity Theft and Fraud: Victims of data breaches face an elevated risk of identity theft and financial fraud. Cybercriminals can exploit exposed personal data, inflicting substantial harm to affected individuals.
Algorithmic Bias
Due to the increase in reliance on AI/ML algorithms in the decision-making process, concerns of potential bias have arisen. Bias in the training data can have significant consequences in financial services as the decisions can affect an individual’s access to credit, investment opportunities, and overall economic well-being.
In the 1930s, Chicago was known for the discriminatory practice of “redlining,” whereby the racial demographics of a given neighborhood heavily determined the creditworthiness of properties. Rumman Chowdhury has reported that Chicago had a history of denying loans to primarily Black neighbourhoods.
In the financial services sector, bias can manifest in various forms, such as racial or gender-based discrimination, socio-economic bias, and other unintended preferences. This can affect:
- Credit decisions: AI bias can severely affect credit scoring. If the historical training data contains biases against certain demographic groups, it results in unfair denial of access to credit or offered less favourable lending terms.
- Investment: AI algorithms can be used to develop investment strategies. Inherent bias can inadvertently favour specific industries, regions, or demographics over others to receive financing.
- Customer service: Chatbots and other AI-powered customer service applications can exhibit interaction biases. For example, chatbots can provide different responses or levels of assistance based on a user’s demographic information, leading to unequal treatment.
Conclusion
Autonomous finance represents a significant shift in the financial industry, leveraging advanced technologies such as AI/ML and blockchain to streamline processes, enhance customer experiences, and drive efficiency. However, implementing autonomous finance has its challenges and considerations.
By addressing these challenges head-on, the financial industry can harness the full potential of autonomous finance to drive sustainable growth – empowering individuals and businesses to achieve their financial goals.